Researchers isolate and decode brain signal patterns for specific behaviors
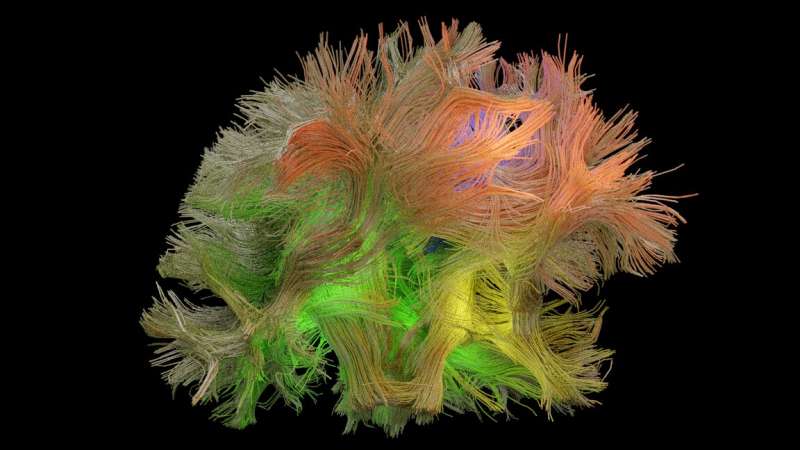
在任何给定的时刻,我们的大脑in various activities. For example, when typing on a keyboard, our brain not only dictates our finger movements but also how thirsty we feel at that time. As a result, brain signals contain dynamic neural patterns that reflect a combination of these activities simultaneously. A standing challenge has been isolating those patterns in brain signals that relate to a specific behavior, such as finger movements. Further, developing brain-machine interfaces (BMIs) that help people with neurological and mental disorders requires the translation of brain signals into a specific behavior, a problem called decoding. This decoding also depends on our ability to isolate neural patterns related to specific behaviors. These neural patterns can be masked by patterns related to other activities and can be missed by standard algorithms.
Led by Maryam Shanechi, Assistant Professor and Viterbi Early Career Chair in Electrical and Computer Engineering at the USC Viterbi School of Engineering, researchers have developed amachine learning algorithmthat resolved the above challenge. The algorithm published inNature Neuroscienceuncovered neural patterns missed by other methods and enhanced the decoding of behaviors that originated from signals in the brain. This algorithm is a significant advance in modeling and decoding of complex brain activity which could both enable new neuroscience discoveries and enhance future brain-machine interfaces.
Standard algorithms, says Shanechi, can miss some neural patterns related to a givenbehaviorthat are masked by patterns related to other functions happening simultaneously. Shanechi and her Ph.D. student Omid Sani developed a machine learning algorithm to resolve this challenge.
Shanechi, the paper's lead senior author says, "We have developed an algorithm that, for the first time, can dissociate the dynamic patterns in brain signals that relate to specific behaviors one is interested in. Our algorithm was also much better at decoding these behaviors from the brain signals."
The researchers showed that their machine learning algorithm can find neural patterns that are missed by other methods. This was because unlike prior methods which only consider brain signals when searching for neural patterns, the new algorithm has the ability to consider both brain signals and the behavioral signals such as the speed of arm movements. Doing so, says Sani, the study's first author, the algorithm discovered the common patterns between the brain and behavioral signals and was also much better able to decode the behavior represented by brain signals. More generally, he adds, the algorithm can model common dynamic patterns between any signals for example, between the signals from different brain regions or signals in other fields beyond neuroscience.
To test the new algorithm, the study's authors, which include Shanechi's Ph.D. students Omid Sani and Hamidreza Abbaspourazad, as well as Bijan Pesaran, Professor of Neural Science at NYU and Yan Wong, a former Post-Doc at NYU, relied on four existing datasets collected in the Pesaran Lab. The datasets were based on recorded changes in the neural activity during the performance of different arm and eye movement tasks.
In the future, this new algorithm could be used to develop enhanced brain-machine interfaces that help paralyzed patients by significantly improving the decoding of movement or speech generated by brain signals and thus translating these signals into a specific, desired behavior such as body movements. This could allow a paralyzed patient to move a robotic arm by merely thinking about the movement or generate speech by just thinking about it. In addition, this algorithm could help patients with intractable mental health conditions such as major depression by separatingbrain signalsrelated tomood symptomsand allowing for real-time tracking of these symptoms (which is outlined in previous studies Shanechi completed). The tracked symptom could then be used as feedback to tailor a therapy to a patient's needs.
Shanechi adds, "By isolating dynamicneural patternsrelevant to different brain functions, this machine learningalgorithmcan help us investigate basic questions aboutbrain's functions and develop enhancedbrain-machine interfacesto restore lost function in neurological and mental disorders."
Explore further
User comments