This article has been reviewed according to Science X'seditorial processandpolicies.Editorshave highlighted the following attributes while ensuring the content's credibility:
fact-checked
peer-reviewed publication
trusted source
proofread
Synthetic data for AI outperform real data in robot-assisted surgery
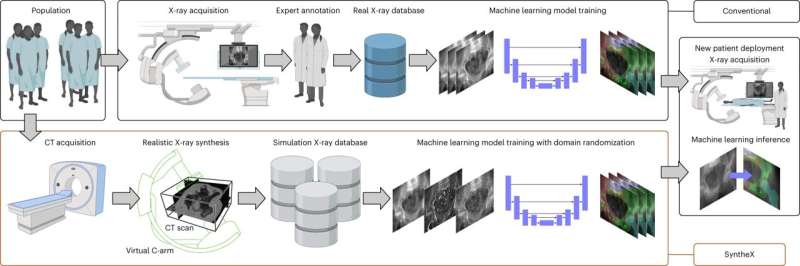
While artificial intelligence continues to transform health care, the tech has an Achilles heel: training AI systems to perform specific tasks requires a great deal of annotated data that engineers sometimes just don't have or cannot get. In a perfect world, researchers would be able to digitally generate the exact data they need when they need it, unlocking new capabilities of AI.
In reality, however, even digitally generating this data is tricky because real-world data, especially in medicine, is complex and multi-faceted. But solutions are in the pipeline. Researchers in the Whiting School of Engineering's Laboratory for Computational Sensing and Robotics have created software to realistically simulate the data necessary for developing AI algorithms that perform important tasks in surgery, such as X-ray image analysis.
The research, published March 20 inNature Machine Intelligence, found that algorithms built with the new system, called SyntheX, could perform as well as or even better than algorithms built from real data in multiple applications, including giving a robot the ability to detectsurgical instrumentsduring procedures.
"We show that generating realistic synthetic data is a viable resource for developingartificial intelligencemodels and much more feasible than collecting real clinical data, which can be incredibly hard to come by or, in some cases, simply doesn't exist yet," said Mathias Unberath, an assistant professor of computer science and senior author of the paper.
Take X-ray guided surgery, for instance. Say you want to develop a new surgical robot and the related algorithms that will allow it to place instruments in the correct places during a procedure. There's just one hitch: The training dataset needed–in this case, highly specific X-ray images–doesn't exist.
The answer? Generate the data needed through simulation, say the researchers. In its study, the team set out to simulate X-ray images that would mirror those taken when a real patient underwent this robot-assisted procedure. To do this, the researchers harnessed the power of sophisticated computer simulations similar to those found in popular simulation video games like The Sims or Minecraft.
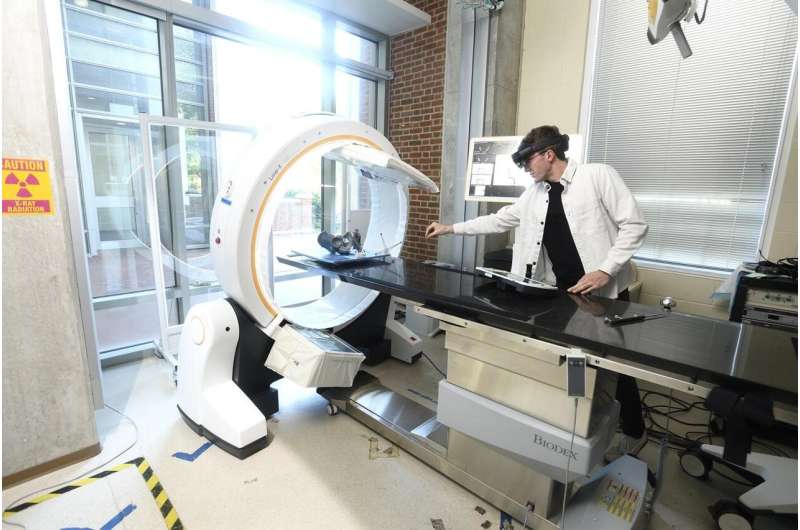
To evaluate precisely just how well simulation-based AI algorithms stack up to those based on real data, the researchers performed a first-of-its-kind study in which they created the same X-ray image dataset both in reality and in their simulation platform.
First, they took a series of real X-rays and CT scans, acquired from cadavers using surgical C-arm X-ray systems. Next, they generated "synthetic" X-ray images that precisely recreated the real-world experiment. Both the real and simulated datasets were then used to develop and train new AI algorithms capable of making clinically meaningful predictions on real X-ray images: hip imaging analysis, robotic surgical instrument detection, and COVID diagnosis. When all was said and done, the team found that the algorithm trained on the simulated data performed as well as thealgorithmtrained on real data.
"Traditionally, models trained on synthetic data don't work well on real clinical data, but that is not the case with SyntheX," said Unberath. "We demonstrated that models trained using only simulated X-rays could be applied to real X-rays from the clinics, without any loss of performance."
The system appears to be one of the first to demonstrate that realistic simulation is both convenient and valuable for developing X-ray image analysis models, which paves the way for all sorts of novel algorithms, the team says.
"Health data, especially for surgery, is a challenge where synthetic data can have a huge impact. Compared to acquiring real patient data, generating large scale simulation data is more flexible, efficient, cheaper, and avoidsprivacy concerns," adds lead-author Cong Gao, a former graduate student in Unberath's lab who is now an image algorithms engineer at Intuitive Surgical.
The team plans to make SyntheX an open-source tool for data simulation, so other researchers can get the datasets they need.
"If you need real data from cadavers or clinics, only very few universities worldwide could do this research. Our system allows researchers to develop meaningful algorithms using onlysimulationand simulated data, which means many more people can meaningfully contribute and innovate in this space," said Unberath.
更多的信息:Cong Gao, Synthetic data accelerates the development of generalizable learning-based algorithms for X-ray image analysis,Nature Machine Intelligence(2023).DOI: 10.1038/s42256-023-00629-1.www.nature.com/articles/s42256-023-00629-1